【機器學習】Bias-Variance, MSE, and Fitting
# 偏差 (BIAS): The difference between Model Output (f(x)) and Real Tag (y)
# 方差 (Variance): The variance of Model Output trained on general dataset (E_D(f_D(x)), 各 f_D(x) 的期望值) and Model Output (f_D(x)) trained on Specific dataset D
# (Model Trained on Specific dataset D) 最小平方差 (Mean Square Error, MSE): (y-f_D(x))^2
---------------------------------------------------------------------------------------------------
經由 Wiki 一連串的推導後,MSE = BIAS^2 + Variance + ϵ^2
# ϵ 為隨機數,為 Real Tag (y) 和 真正的 function (f) 的 output 的差,詳見 Wiki
也因此,一個好的模型 (Low MSE) 不能 Overfitting 或 Underfitting
Overfitting causes BIG Variance; Underfitting causes BIG BIAS.
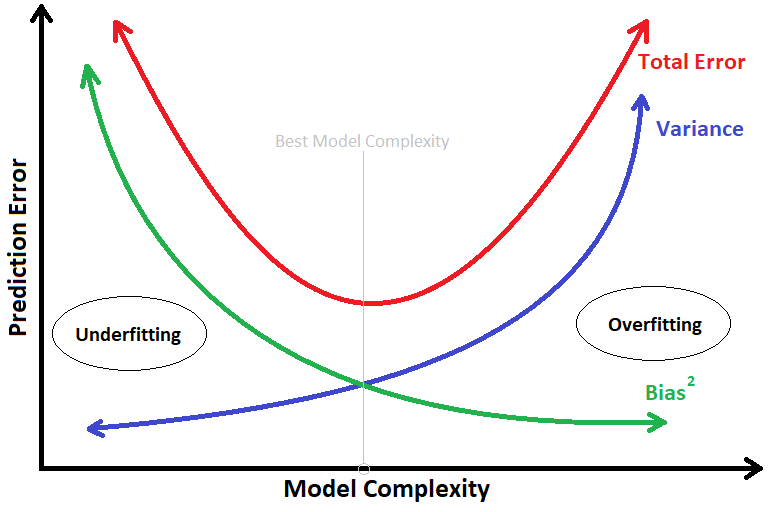
Reference: