How Can Generative AI Development Power Predictive Analytics?
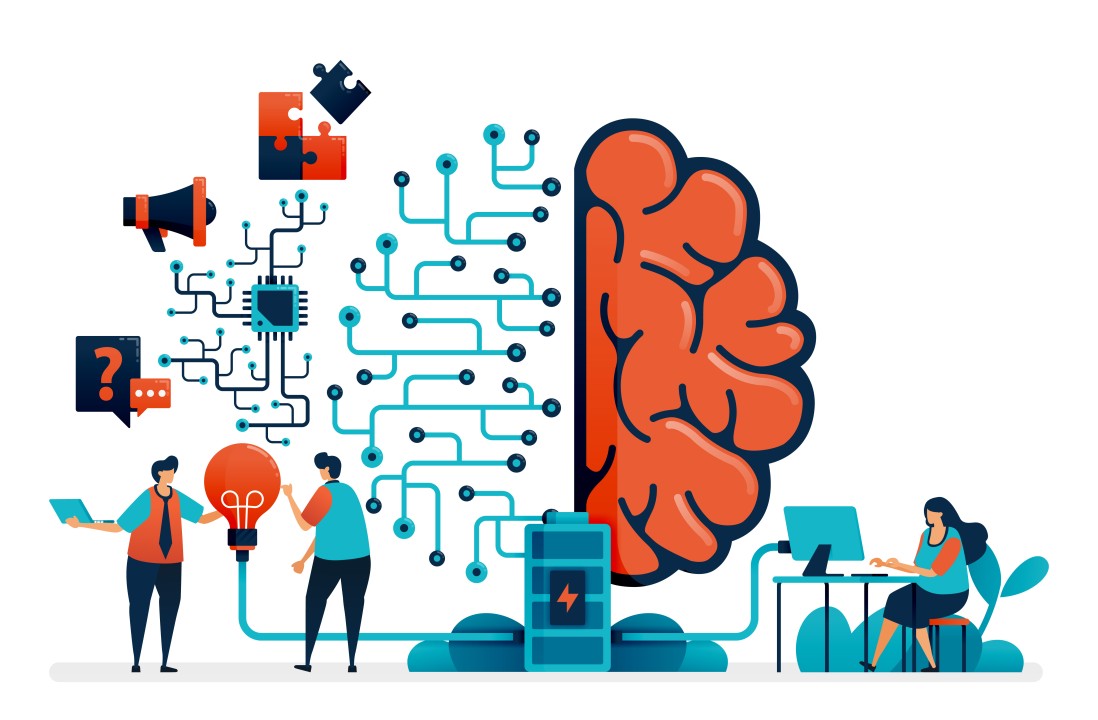
Predictive analytics has emerged as a transformative force across various industries, enabling organizations to make data-driven decisions and forecast future trends. At the forefront of this evolution is generative artificial intelligence (AI), a powerful technology that is reshaping how businesses analyze data and derive insights. This blog explores how generative AI development can enhance predictive analytics, offering businesses a significant competitive advantage.
Understanding Generative AI and Predictive Analytics
What is Generative AI?
Generative AI refers to algorithms that can create new content, including text, images, audio, and more, by learning patterns from existing data. Unlike traditional AI models that primarily classify or analyze data, generative AI can generate new data points, providing a richer understanding of potential future scenarios. Examples include natural language processing models like GPT-4 and image synthesis tools like DALL-E.
What is Predictive Analytics?
Predictive analytics is a branch of advanced analytics that uses statistical algorithms and machine learning techniques to identify the likelihood of future outcomes based on historical data. By employing techniques such as regression analysis, time series analysis, and machine learning, businesses can forecast trends, optimize operations, and enhance decision-making.
The Synergy Between Generative AI and Predictive Analytics
The integration of generative AI into predictive analytics is a game-changer, as it can enhance the predictive capabilities of traditional analytics models in several ways:
1. Enhanced Data Generation
One of the primary benefits of generative AI is its ability to create synthetic data. In many scenarios, organizations may lack sufficient historical data to make accurate predictions. Generative AI can produce realistic synthetic datasets that mimic real-world data, allowing predictive models to train on larger and more diverse datasets.
Example:
A healthcare organization may have limited patient data for a specific condition. By using generative AI to create synthetic patient records, the organization can develop predictive models to identify risk factors and potential treatment outcomes more accurately.
2. Improved Feature Engineering
Generative AI can automate feature engineering, a critical step in predictive analytics where relevant variables are identified and created from raw data. By learning the underlying patterns in the data, generative models can suggest or create new features that enhance the predictive power of traditional models.
Example:
In finance, a generative AI model analyzing transaction data may identify hidden features that correlate with fraud risk. These new features can be integrated into fraud detection models, improving their accuracy.
3. Scenario Simulation and Forecasting
Generative AI enables businesses to simulate various scenarios, allowing them to explore different outcomes based on changing inputs. This capability enhances predictive analytics by providing a broader range of potential future scenarios, helping organizations prepare for uncertainty.
Example:
Retailers can use generative AI to simulate various inventory management scenarios based on changing consumer behavior, seasonal trends, and economic conditions. This helps them optimize stock levels and reduce costs.
4. Better Handling of Missing Data
Missing data is a common challenge in predictive analytics. Generative AI can help fill in gaps in datasets by generating plausible values for missing entries based on learned patterns. This capability allows for more accurate predictions and reduces biases that arise from incomplete data.
Example:
In a survey dataset where some respondents did not answer specific questions, generative AI can estimate reasonable responses based on the available data, ensuring that predictive models are not skewed by missing information.
5. Real-Time Data Analysis
Generative AI can process and analyze data in real-time, enabling organizations to make timely decisions. This is particularly valuable in industries where conditions change rapidly, such as finance and e-commerce.
Example:
In stock trading, generative AI can analyze market trends and generate predictive insights in real-time, allowing traders to act swiftly on emerging opportunities or risks.
Case Studies: Generative AI in Predictive Analytics
1. Marketing Campaign Optimization
A leading e-commerce company employed generative AI to enhance its marketing campaign strategies. By generating synthetic customer profiles and simulating responses to different promotional strategies, the company could predict which campaigns would yield the highest engagement and sales. As a result, they increased their return on investment by 30% through more targeted marketing efforts.
2. Predictive Maintenance in Manufacturing
A manufacturing firm integrated generative AI into its predictive maintenance strategies. By analyzing machine data and generating simulated failure scenarios, the company could predict equipment failures before they occurred. This proactive approach reduced downtime by 40% and saved significant maintenance costs.
3. Healthcare Predictive Modeling
In the healthcare sector, a hospital utilized generative AI to create synthetic patient records to train predictive models for patient readmission risks. The insights gained from these models enabled the hospital to implement targeted intervention strategies, reducing readmission rates by 25%.
Challenges and Considerations
While the integration of generative AI into predictive analytics offers numerous benefits, it also presents challenges that organizations must navigate:
1. Data Quality and Bias
The effectiveness of generative AI models depends heavily on the quality of the data used for training. If the training data contains biases, the generated data and predictions may also reflect those biases. Organizations must ensure that their datasets are diverse and representative to mitigate this risk.
2. Complexity of Implementation
Integrating generative AI into existing predictive analytics frameworks can be complex. Organizations may require specialized skills and resources to develop and implement generative AI models effectively.
3. Ethical Considerations
The use of generative AI raises ethical questions, particularly regarding data privacy and the potential misuse of generated content. Organizations must prioritize ethical considerations and establish guidelines to ensure responsible use of generative AI.
The Future of Generative AI in Predictive Analytics
The future of generative AI in predictive analytics looks promising, with advancements in AI technology paving the way for more sophisticated models. As organizations increasingly adopt generative AI, we can expect:
Improved Collaboration Between AI and Human Analysts: Generative AI will augment the capabilities of human analysts, enabling them to focus on high-level insights while the AI handles data generation and feature engineering.
Advancements in Explainability: As generative AI models become more transparent, organizations will better understand how predictions are made, fostering trust in AI-driven insights.
Wider Adoption Across Industries: The benefits of generative AI in predictive analytics will lead to broader adoption across sectors such as finance, healthcare, retail, and manufacturing.
Conclusion
Generative AI development has the potential to revolutionize predictive analytics by enhancing data generation, improving feature engineering, enabling scenario simulation, and providing real-time insights. As businesses continue to embrace this technology, they can gain a competitive edge, make informed decisions, and navigate uncertainties more effectively. However, organizations must also be mindful of the challenges and ethical considerations associated with generative AI, ensuring responsible and effective implementation. The synergy between generative AI and predictive analytics is not just a trend but a significant step toward smarter, data-driven decision-making in the future.