Synthetic Data: When You Can't Get the Real Deal, Grow Your Own Damn Data
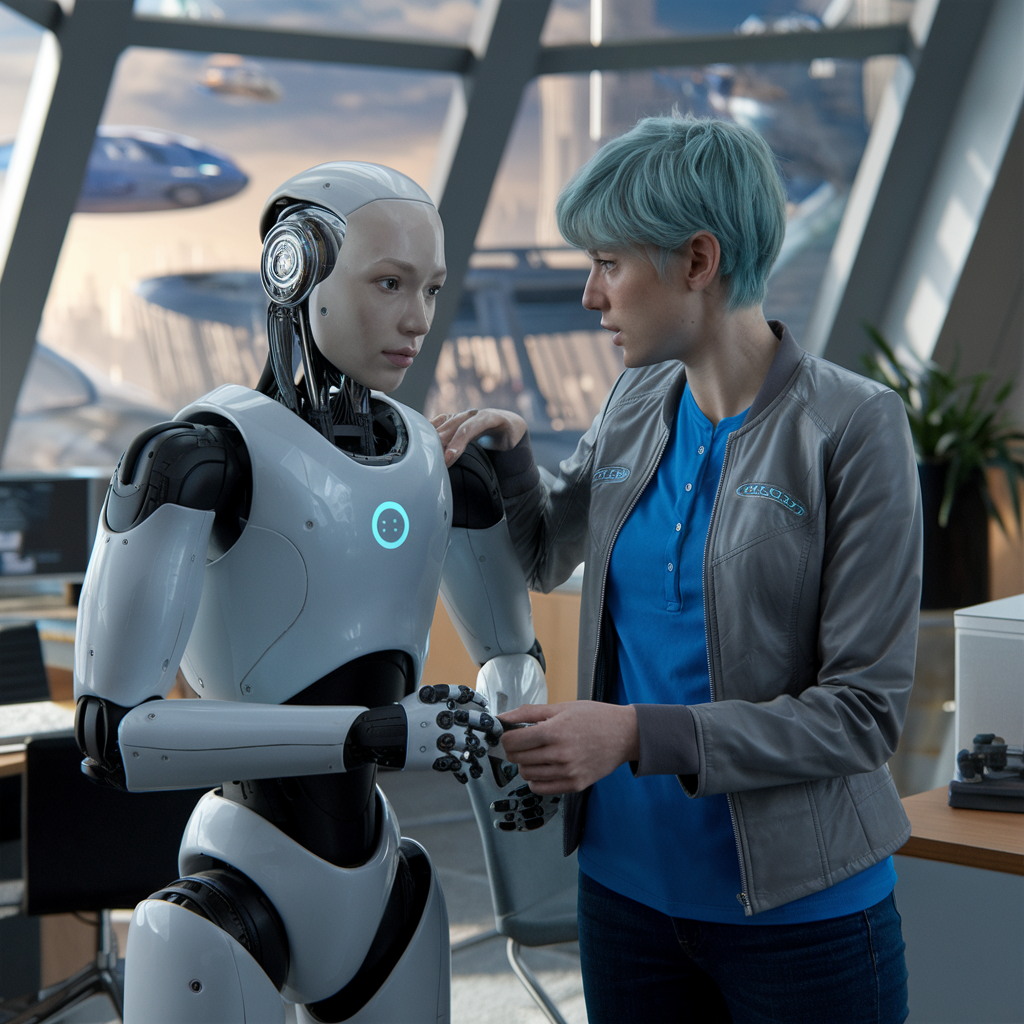
Hold on to a nanosecond. Training AI models on real-world data is becoming a bottleneck faster than a Cybertruck in a tunnel. The problem? Real data is messy, biased, expensive to acquire, and riddled with privacy nightmares. Enter synthetic data — the clever engineering workaround that’s got tech companies acting like digital farmers.
Think of it like this: You need to teach an AI to recognize cats. Instead of painstakingly labeling millions of actual cat photos (and dealing with feline-related lawsuits, probably), you algorithmically generate a gazillion virtual cats. Different breeds, poses, environments — the whole shebang, created in a digital sandbox.
Why the sudden shift to digital data farms? A few brutally logical reasons:
Privacy? Problem Solved (Mostly): Synthetic data isn’t tied to real individuals, meaning less ethical baggage and fewer regulatory headaches. It’s like teaching a robot to identify criminals without ever showing it a real mugshot. Clever, right?
No More Data Bias Nightmares (Theoretically): You can design synthetic datasets to be perfectly balanced, mitigating the biases that plague real-world data and lead to discriminatory AI. Of course, the human factor in designing these synthetic datasets still needs scrutiny — garbage in, garbage out, even if it’s artificially generated garbage.
Endless Supply, On Demand: Need more training data for a rare edge case? Just crank up the synthetic data generator. Forget the limitations of the real world; you’re building your own damn data universe.
Speed & Efficiency: Generating synthetic data is often faster and cheaper than acquiring and labeling real data. Time is money, especially when you’re racing to build the next breakthrough AI.
But before we declare real data obsolete, a dose of reality:
The Fidelity Factor: How closely does synthetic data mirror the complexities and nuances of the real world? If your virtual cats don’t behave like actual cats, your AI might be in for a shock when it encounters a real feline.
The “Unknown Unknowns”: Real-world data often throws curveballs — unexpected patterns and edge cases that synthetic data might miss. You can’t simulate everything.
The Bottom Line:
Synthetic data isn’t a panacea, but it’s a damn clever tool for overcoming some of the major roadblocks in AI development. Expect to see more companies embracing this approach — generating their own training data universes to accelerate progress and sidestep the limitations of reality. It’s like creating the Matrix to train the machines… but hopefully with fewer red pills and existential dread. Let’s see if they can make it work.