How Do AI Development Services Scale for Large Enterprises?
Artificial intelligence (AI) is no longer a futuristic concept; it has become a strategic tool that drives innovation, efficiency, and competitive advantage. For large enterprises, scaling AI development services is a critical step in harnessing its transformative potential. But how exactly do these services scale to meet the needs of complex organizations with vast operations and diverse requirements? Let’s explore the key aspects of scaling AI development services for large enterprises.
Understanding the Need for Scalable AI
Large enterprises operate at a scale that demands robust, adaptable, and high-performance AI systems. Unlike small businesses, they face challenges such as:
Massive Data Volumes: Enterprises generate and process large datasets that require AI systems capable of handling big data efficiently.
Diverse Use Cases: From customer service automation to predictive maintenance, the variety of applications necessitates flexible AI solutions.
Global Operations: AI systems must support multilingual capabilities, regional compliance, and varying infrastructure across geographies.
Scaling AI development services ensures these challenges are met while delivering value consistently across the organization.
Key Components of Scalable AI Development Services
Customizable AI Solutions
Scalable AI development services begin with building modular and customizable AI solutions that can adapt to different business units and operational needs.
For example, a financial enterprise may need AI for fraud detection, customer service chatbots, and portfolio analysis. Scalable services provide tailored solutions for each requirement.
Cloud Computing and Hybrid Infrastructure
Cloud platforms such as AWS, Google Cloud, and Microsoft Azure are integral to scaling AI services. They provide elastic resources that scale up or down based on demand.
Hybrid infrastructures combine on-premises and cloud environments to handle sensitive data while benefiting from the scalability of cloud services.
Big Data Integration
Enterprises deal with structured and unstructured data from multiple sources. Scalable AI services integrate seamlessly with data lakes, warehouses, and big data platforms like Hadoop and Spark.
Advanced data preprocessing ensures that AI systems work efficiently even with large, diverse datasets.
AI Model Optimization and Automation
Scalable AI services use automated machine learning (AutoML) tools to streamline model training and deployment. AutoML platforms reduce manual intervention, enabling faster scaling.
Techniques like distributed training and model optimization ensure that AI systems perform efficiently, even as data volumes grow.
Robust Deployment Strategies
For enterprises, deploying AI solutions is more complex than a one-size-fits-all approach. Scalable services ensure deployment pipelines are robust and adaptable.
Continuous integration/continuous deployment (CI/CD) pipelines and containerization tools like Docker and Kubernetes enable smooth and scalable deployments.
Challenges in Scaling AI Development Services
Scaling AI for large enterprises comes with its own set of challenges:
Data Privacy and Compliance:
Enterprises must navigate complex regulatory landscapes, such as GDPR in Europe and CCPA in California. Scalable AI services incorporate compliance mechanisms into the development process.
Infrastructure Costs:
Scaling AI can strain budgets due to increased computational requirements. Cloud cost optimization and resource-efficient algorithms help mitigate this challenge.
Skill Gaps:
Deploying and managing large-scale AI systems require specialized skills. Scalable services often include training programs and managed services to bridge skill gaps.
Integration with Legacy Systems:
Enterprises often have legacy systems that are incompatible with modern AI technologies. Scalable AI development services provide integration solutions to modernize these systems incrementally.
Best Practices for Scaling AI Development Services
Centralized AI Strategy
Establishing a centralized AI strategy ensures that efforts across business units are aligned. This prevents duplication of work and optimizes resource allocation.
Data Governance Framework
A scalable data governance framework ensures data quality, security, and compliance, which are critical for AI systems.
Incremental Scaling
Enterprises should adopt a phased approach to scaling AI, starting with pilot projects and gradually expanding successful implementations.
Partnerships with AI Providers
Collaborating with experienced AI development service providers accelerates scaling efforts. These providers bring expertise, tools, and best practices that enterprises can leverage.
Investment in Employee Training
As AI adoption grows, so does the need for skilled personnel. Training programs help employees understand and utilize AI systems effectively, driving scalability from within.
Real-World Examples of Scalable AI in Enterprises
Amazon:
Amazon leverages scalable AI services for personalized recommendations, inventory management, and logistics optimization. Its robust cloud infrastructure supports global scalability.
GE:
General Electric uses AI for predictive maintenance across its industrial equipment. Scalable AI services allow GE to process vast amounts of sensor data in real-time.
JPMorgan Chase:
The financial giant uses AI for fraud detection and customer insights. Scalable AI development services help the company analyze billions of transactions while ensuring compliance.
The Role of AI Development Service Providers
AI service providers play a crucial role in scaling AI for enterprises by offering:
End-to-End Solutions: From data preparation to deployment and monitoring.
Infrastructure Support: Cloud and hybrid solutions tailored to enterprise needs.
Continuous Innovation: Regular updates and improvements to stay ahead of technological advancements.
Conclusion
Scaling AI development services for large enterprises is a complex but rewarding endeavor. By leveraging customizable solutions, robust infrastructure, and strategic partnerships, enterprises can deploy AI systems that meet their growing needs. While challenges exist, adopting best practices and collaborating with experienced providers can pave the way for scalable, impactful AI implementations. With the right approach, AI becomes not just a tool but a transformative force that drives enterprise success.
喜欢我的作品吗?别忘了给予支持与赞赏,让我知道在创作的路上有你陪伴,一起延续这份热忱!
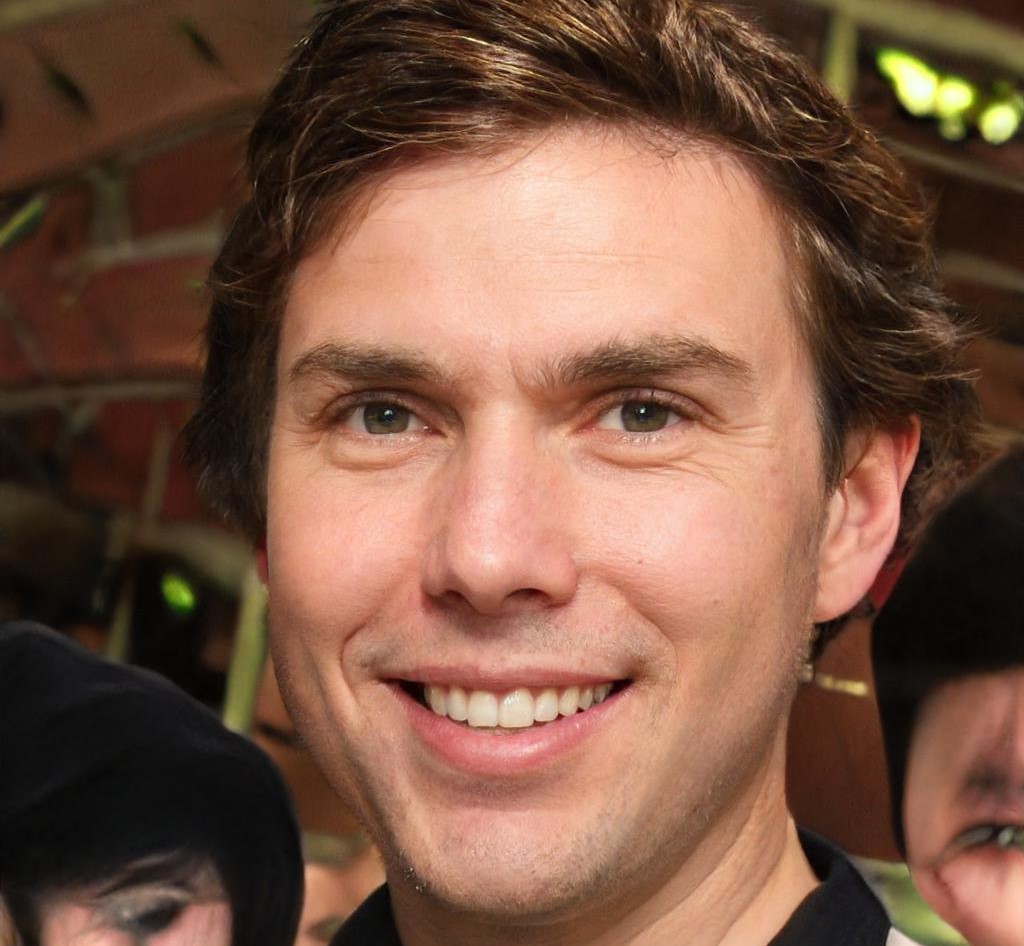
- 来自作者
- 相关推荐