How Do Custom Large Language Models Drive Innovation in AI?
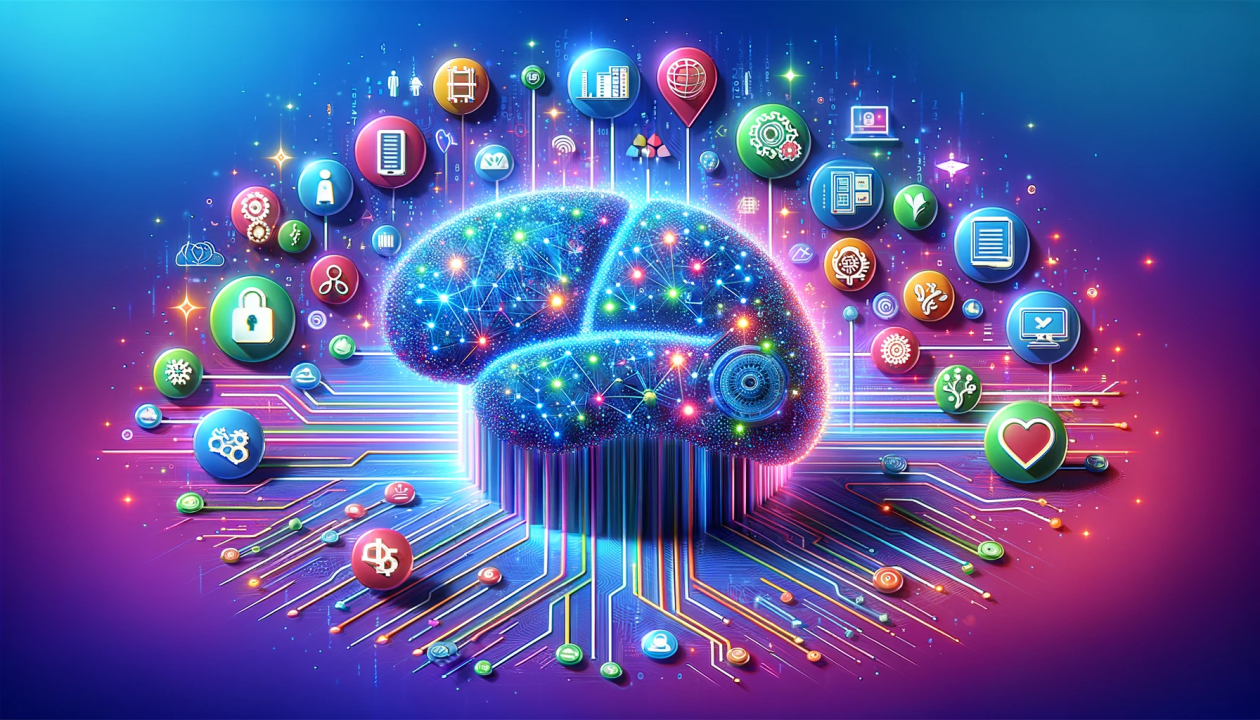
Artificial intelligence (AI) has undergone a revolutionary transformation in the past decade, largely due to the rise of large language models (LLMs). These models, such as GPT-3 and its successors, have demonstrated impressive capabilities in natural language understanding and generation, making them integral to various sectors. However, beyond the well-known models like GPT, custom large language models (custom LLMs) have emerged as a powerful tool for driving innovation in AI. In this blog post, we will explore how custom LLMs contribute to AI advancement, their benefits, applications, and their role in the future of AI.
What are Custom Large Language Models?
Large Language Models (LLMs) are a class of deep learning models trained on vast datasets to understand and generate human-like text. These models, often based on transformer architectures, are designed to learn patterns in language, including grammar, context, and semantics, from large text corpora. Examples of such models include OpenAI's GPT-3, Google's BERT, and Meta's LLaMA.
Custom large language models, as the name suggests, are tailored versions of these models to meet specific needs or challenges of particular industries, companies, or applications. Instead of using a general-purpose model, custom LLMs are fine-tuned on niche datasets or designed with specific goals in mind, such as enhancing customer service, improving content generation, or accelerating research in specialized fields.
The Role of Custom LLMs in AI Innovation
Custom LLMs are helping to shape the future of AI in several transformative ways. Below are some key areas where these models are driving innovation:
1. Industry-Specific Applications
One of the most significant contributions of custom LLMs is their ability to solve problems unique to specific industries. By training a model on data relevant to an industry, companies can create LLMs that are not only proficient in language generation but also deeply knowledgeable about industry-specific terminology, practices, and challenges.
For example:
Healthcare: In healthcare, a custom LLM could be trained on medical literature, clinical notes, and research papers. This would enable the model to understand medical terminology and assist in tasks such as diagnosing diseases, suggesting treatments, or aiding in medical research.
Legal: A legal firm could build a custom LLM trained on legal texts, case law, and court documents. This could help in drafting legal documents, conducting research, and even automating some aspects of legal consultation.
Finance: In finance, custom LLMs can be trained on financial reports, market analysis, and economic data, providing accurate insights into investment opportunities, risk assessments, and market predictions.
These specialized models not only enhance the precision of outputs but also save time and resources by reducing the need for human expertise in these niche areas.
2. Improved Accuracy Through Domain-Specific Training
While general-purpose LLMs are extremely powerful, they are not perfect for all scenarios. General models are trained on diverse data sources, meaning they may not perform optimally in specialized fields where domain-specific knowledge is crucial. Custom LLMs can be fine-tuned to address this limitation.
For instance, when trained with a focused dataset, a custom LLM can significantly improve its accuracy in understanding and generating text related to a particular domain. This is especially useful in tasks such as:
Technical Support: A custom LLM for tech support could be trained on troubleshooting guides and previous customer queries. This would enable it to provide faster and more accurate responses to users, enhancing the customer experience.
Customer Interaction: Brands can build custom LLMs to engage with customers in a more personalized manner. These models can handle queries specific to a company’s products or services, creating a more seamless and efficient customer support system.
By training on specific, relevant data, custom LLMs deliver higher-quality, context-aware outputs.
3. Efficiency Gains and Cost Reduction
Custom LLMs drive innovation by enabling organizations to automate and streamline their processes. In many cases, businesses that rely on large-scale data processing and text generation can significantly reduce costs by deploying custom-trained models to handle repetitive tasks.
For example:
Content Generation: Custom LLMs can be trained to produce high-quality content that fits a company’s voice and style. Whether it's generating blog posts, social media updates, or marketing materials, a well-trained custom model can create relevant content at scale, saving both time and resources.
Data Analysis: Businesses in data-heavy industries like e-commerce, logistics, or manufacturing can create custom LLMs that automate the analysis of large volumes of text data. Whether it’s customer reviews, product descriptions, or even employee feedback, custom LLMs can uncover patterns and insights that drive decision-making.
By automating mundane tasks, businesses can reallocate human resources to more strategic activities, improving overall productivity.
4. Enhanced Personalization
One of the most exciting aspects of custom LLMs is their ability to create highly personalized user experiences. These models can be fine-tuned to understand individual user preferences, behaviors, and patterns, leading to more tailored interactions.
For example:
E-commerce: A custom LLM trained on past customer interactions, product reviews, and purchase history can help create a personalized shopping experience. By suggesting relevant products or answering customer queries in a more engaging and relevant way, the model enhances user satisfaction and sales.
Education: In the education sector, custom LLMs can be trained on specific teaching materials and student data to offer personalized learning experiences. They can adapt to a student’s learning pace, recommend resources, and even provide explanations in a manner that suits the student’s learning style.
This level of personalization fosters a deeper connection between users and the product, resulting in improved engagement and loyalty.
5. Fostering Cross-Industry Innovation
As custom LLMs become more sophisticated, their applications are transcending industry boundaries. The ability to create models that can operate across various sectors has the potential to spark cross-industry innovation. For example, a custom LLM developed in the healthcare sector could be adapted for use in life sciences, pharma, or public health research.
This cross-pollination of ideas helps drive innovation, particularly when models trained in one domain can provide insights or solutions to problems in an entirely different industry. For instance:
Collaboration Between Healthcare and AI: AI-driven custom LLMs are playing a vital role in creating smarter health diagnostics, combining machine learning with data from medical research, clinical trials, and patient records to improve patient care.
Environmental Sustainability: Custom LLMs trained on environmental data can generate insights to improve sustainability practices across industries, from energy to manufacturing, contributing to better environmental decision-making.
This collaborative innovation, powered by custom LLMs, accelerates the development of novel solutions to complex global challenges.
Challenges in Custom LLM Development
While custom LLMs are undoubtedly driving AI innovation, their development comes with its own set of challenges. Key hurdles include:
Data Privacy and Security: Collecting and handling sensitive data, especially in fields like healthcare and finance, raises concerns about data privacy and security. Companies must adhere to strict regulations such as GDPR or HIPAA when training custom LLMs on private data.
Bias and Fairness: LLMs are only as good as the data they are trained on. If the training data is biased, the resulting model will likely reflect those biases, which could have ethical implications, especially in sensitive applications like recruitment or law enforcement.
Computational Costs: Training large models requires significant computational resources, which can be expensive. Companies must ensure they have the necessary infrastructure or rely on cloud services that provide scalable solutions for model training.
Conclusion
Custom large language models are driving unprecedented innovation in AI by enabling the creation of tailored solutions for specific industries, improving accuracy and efficiency, and fostering deeper personalization. Their versatility is opening up new possibilities in fields ranging from healthcare to finance, creating smarter, more efficient systems. As the technology continues to advance, the future of AI will increasingly be shaped by custom LLMs that provide greater specialization, cross-industry collaboration, and more impactful, data-driven decision-making.
The next frontier for custom LLMs lies in addressing the challenges associated with their development, particularly in areas of bias, security, and computational costs. However, the potential of custom LLMs to unlock innovative solutions in AI remains vast, and we are only beginning to scratch the surface of what is possible. With ongoing research and development, custom large language models will continue to revolutionize industries and drive the future of AI forward.
喜欢我的作品吗?别忘了给予支持与赞赏,让我知道在创作的路上有你陪伴,一起延续这份热忱!